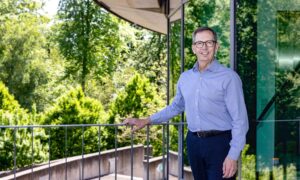
Welcome: Noel O’Boyle
EMBL-EBI’s new Chemical Biology Resources Team Leader aims to encourage community engagement and explore AI-driven approaches
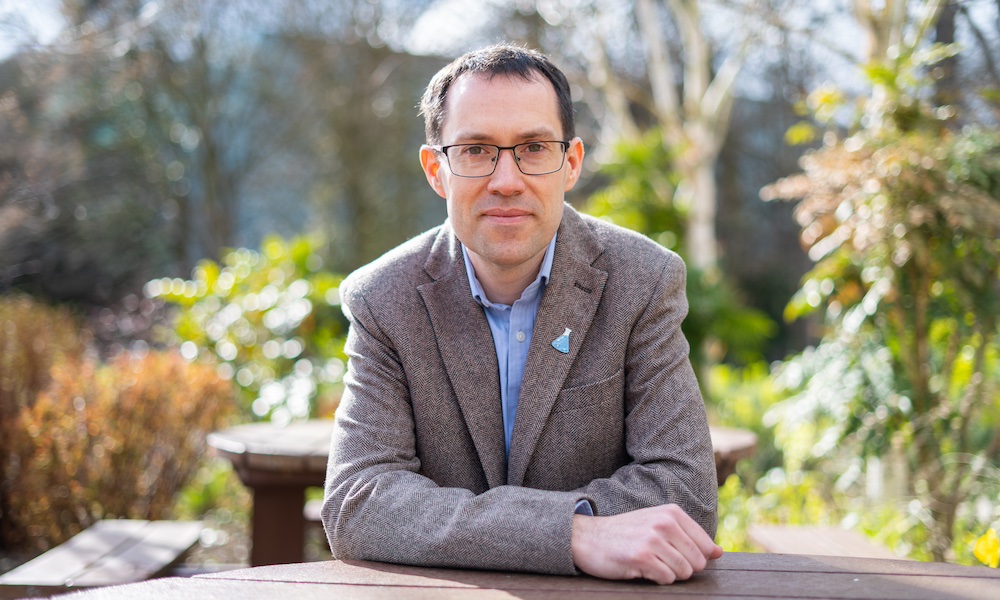
The Chemical Biology Resources Team at EMBL-EBI is responsible for curating, maintaining, and developing critical open data resources that provide high-quality chemical data. Researchers in academia and the pharmaceutical industry use these resources to accelerate drug discovery and scientific research.
Noel O’Boyle has joined EMBL-EBI as the new Chemical Biology Resources Team Leader. We spoke to O’Boyle about his journey from chemistry to cheminformatics, his thoughts on enhancing chemical biology resources using AI, and his plans for the team.
What is your professional background?
I earned a degree in chemistry followed by a PhD in inorganic chemistry. During my doctoral research, I became increasingly drawn to computational methods and programming, interests I’ve held since childhood. After my PhD, I undertook postdoctoral roles in computational drug discovery at University College Dublin, and subsequently at the Cambridge Crystallographic Data Centre and University College Cork.
Eventually, I moved back to Cambridge, first joining the cheminformatics startup NextMove Software and then transitioning to Nxera Pharma, where I led the Cheminformatics team.
Can you please explain what the Chemical Biology Resources team does, in simple terms?
Our team curates and maintains EMBL-EBI’s chemical biology data resources. We collect information about small molecules, their structures, and how they interact with biological targets. We then ensure these data are accurate, consistent, and easy to navigate for our users. The team also develops tools and platforms that allow researchers to make use of this information for drug discovery and more.
Can you tell us more about EMBL-EBI’s chemistry data resources?
ChEMBL is a large-scale database of bioactive molecules with drug-like properties. In ChEMBL, you can find the chemical structures of these molecules and the results of biological assays reported in the scientific literature. Scientists rely on ChEMBL to identify potential drug candidates, understand how different chemical compounds perform in various assays, and compare new compounds against known data to guide experiments or trials.
SureChEMBL extracts chemical information from patent literature. Patents often contain detailed synthetic routes – the sequences of chemical reactions and conditions used to create a drug molecule – and compounds that may not appear in other literature. Patent literature can also be very long and difficult to interpret. SureChEMBL automates the process of parsing patents, making it easier for researchers to see if a given compound has been patented. This helps to identify patent infringement risks and avoid duplicating efforts.
UniChem acts as a unifying service that cross-links information about small molecules across different databases, including those managed by EMBL-EBI and external databases such as PubChem and Mcule. By providing consistent identifiers, UniChem allows users to move between databases and avoid confusion caused by different naming conventions or database IDs.
ChEBI provides curated information on the definitions and relationships of small chemical compounds. Many biologically related databases use identifiers from ChEBI for chemical compounds. Researchers use ChEBI to find standardised chemical terminology and ensure consistent annotation of chemical compounds when writing scientific manuscripts.
How do you think AI is changing the data resources your team manages?
AI and machine learning rely on large, high-quality data sets, and this is precisely what our resources provide. Researchers worldwide can leverage these resources to build predictive models to help identify promising drug candidates.
Ongoing advances in AI have highlighted two major opportunities for our team. Firstly, AI can enhance our curation efforts. Using text mining and natural language processing to identify key data in articles or patents can help our curators focus on the most relevant information and speed up data entry.
Secondly, AI can be used to improve our search interfaces. This would let us move beyond traditional keyword-based searches and toward natural language queries, where researchers can ask a question and retrieve curated information in a more intuitive manner. This is something we are currently working towards.
What are some of the first things you’re hoping to do in your new role?
One priority is strengthening our user community. I’d like to organise a community meeting where users, curators, developers, and researchers share needs, challenges, and wishlists. I would also like to expand coverage in emerging areas like biologics – therapeutics like peptides, oligosaccharides, oligonucleotides, antibodies – to better reflect the industry’s shift toward new therapeutic modalities.
How do you collaborate with other teams across EMBL-EBI?
We regularly work with neighbouring teams, including the Protein Data Bank in Europe (PDBe) team. By linking protein structure data to small-molecule information in ChEMBL, we help researchers understand protein–ligand interactions. We also have collaborations with Open Targets to leverage ChEMBL’s data to help prioritise and validate targets for drug discovery.
Can you tell us about some of your hobbies and interests?
I enjoy playing badminton, exploring the Cambridgeshire countryside, and playing music on a variety of instruments. In fact, a few years ago when I purchased a second-hand piano, I was able to combine two of my interests – music and chemistry (see photo).
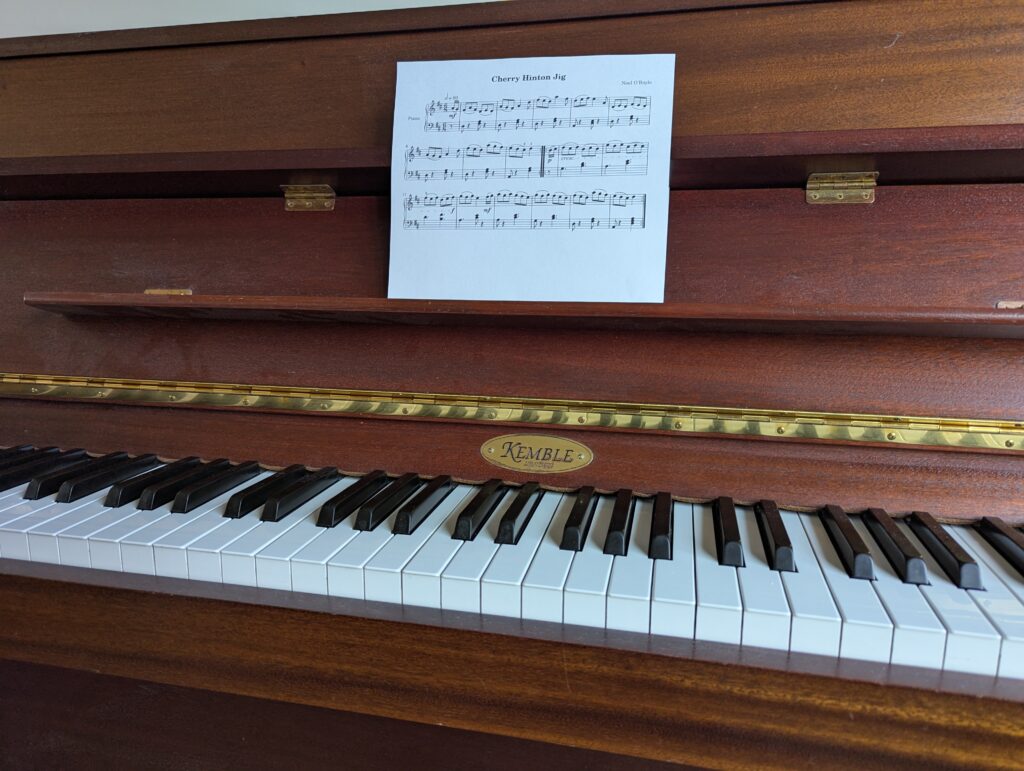