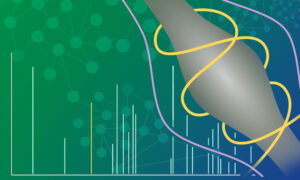
Read the latest Issue
How text mining collaborations benefit our research, data resources, and the wider scientific community
Text mining is the process of analysing vast amounts of textual material to extract meaningful concepts, relationships, and trends using machine learning approaches. It enables researchers to rapidly find new and hidden information in text-based sources. When these techniques are applied to scientific publications, it becomes possible to uncover new meaning and hidden patterns that would otherwise take years to manually curate.
Tackling data challenges and ensuring that we are able to exploit large datasets to their full potential for life science research is a key part of the Data Sciences Plans within EMBL’s Molecules to Ecosystems Programme. This includes developing and experimenting with new technologies and machine learning approaches. For example, these methods are used in a variety of projects to extract new information from publications. This includes mining and extraction of gene–disease associations for drug discovery, enriching our services with metagenomics data, and providing information to the wider text mining community to help others train their own machine learning algorithms.
Europe PMC is EMBL-EBI’s open science platform for life science publications. It’s available to anyone, anywhere for free. With Europe PMC, scientists can search and read over 40 million publications, preprints, and other documents enriched with links to supporting data, protocols, etc.
Text mining approaches are hugely beneficial for improving the way we identify novel drug targets. A vast amount of information on gene–disease associations and associated drug targets already exists online, hidden within millions of scientific publications. Manually sorting through these texts would take decades. However, using text mining to search the literature allows data to be accessed and analysed for more rapid drug discovery.
In collaboration with Open Targets, researchers at Europe PMC are doing just this by creating a pipeline that maximises literature information extraction using named entity recognition (NER) models. Named Entity Recognition (NER) is a widely used natural language processing approach to identify real-world objects, such as people, location, and time within text. The Europe PMC team uses this approach to identify genes, proteins, diseases, chemicals, and other biomedical concepts from life science literature. These bioNERs form the basis of gene–disease association identification from literature for Open Targets.
NER models are a form of natural language processing (NLP) – a type of machine learning method which allows computers to analyse text rather than computer code. In this case, the natural language being detected consists of disease and gene terms found within life science literature.
“For our machine learning algorithms to work effectively we needed to train them with high-quality data,” said Shyamasree Saha, Machine Learning and Text Mining Scientist at EMBL-EBI. “At Europe PMC, we developed a gold standard dataset for genes, proteins, disease, and organisms. We are using BioBERT, a domain-specific language model pre-trained on a large biomedical corpora and fine-tuning the model for the NER task using our gold standard dataset. The model replaces our old dictionary based NER approach and significantly improves entity association identification accuracy.”
Learn more about how NER is being used to develop the Open Targets Platform.
Metadata – the information that describes where, when, and how specific data are obtained – enriches the scientific value of genomic sequencing data and makes data FAIR (Findable, Accessible, Interoperable, and Reproducible). However, these metadata are frequently missing from databases or contain poor quality descriptions, meaning they cannot be used to interpret the data. For metagenomics – the direct analysis of genomes contained within an environmental sample – the use of metadata is of vital importance to increase data reuse and improve interpretation.
Researchers from Europe PMC and EMBL-EBI’s metagenomics data resource MGnify, have found a solution to this challenge by automatically extracting relevant metadata key terms straight from the literature. This is done using a machine learning framework to mine a wide range of metagenomics studies found in publications stored within the Europe PMC database. The project is called Enriching MEtagenomics Results using Artificial intelligence and Literature Data (EMERALD).
“One of the major limitations when comparing datasets is the lack of contextual metadata relating to a sample,” said Lorna Richardson, Coordinator for MGnify at EMBL-EBI. “To address this, we partnered with Europe PMC to automatically extract relevant metadata terms from publications, improving the range and depth of metadata available to our users. This metadata includes terms relating to the sequencing platform used, extraction kits, primers, the environment of the sample, and much more, which will help researchers get the most out of the data stored in MGnify.”
Find out more about how the EMERALD project is benefiting MGnify users.
Finally, the Europe PMC database itself is helping to advance the field of text mining by simplifying the way its users can find and access data from scientific literature. One of the tools available within Europe PMC is the annotation tool. This allows users developing their own text mining algorithms to quickly extract relevant terms and use them to develop their own text mining pipelines.
The annotations within this tool are collected by both Europe PMC and the wider text mining community and they include biological terms such as disease names, chemicals, and proteins. The annotation terms available for each article are located in the tools menu within Europe PMC and can also be accessed programmatically using the annotations API.
“We have close to 1.6 billion annotations available to help our users locate entities in the full text and abstracts of articles stored in Europe PMC,” said Aravind Venkatesan, Senior Data Scientist at EMBL-EBI. “These are available through the Europe PMC annotations tool, which supports scientists and database curators in their literature research by making it easy to find the relevant annotation terms they need to train their text mining models. This will help advance a range of research fields and also accelerate the field of text mining itself.”
Text mining is a tool which can benefit many research areas by increasing the rate at which we can unlock uncharted information already present in the millions of life science articles published online. Here we have shown how EMBL-EBI scientists have been able to harness the power of text mining to accelerate fields including drug discovery and metagenomics research. But it doesn’t stop there; this same approach can be used to leverage a vast range of fields with endless possibilities. Text mining to advance the life sciences is still a young field, but it is an exciting one to be a part of right now.
Find out more about the Data Sciences Plans at EMBL.
The EMERALD project is funded by the UK Research and Innovation (UKRI).
Open Targets funding.
Looking for past print editions of EMBLetc.? Browse our archive, going back 20 years.
EMBLetc. archive