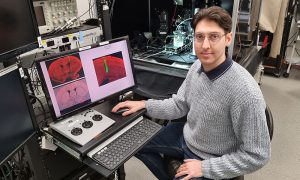
Read the latest Issue
Experience in data management and coordination has enabled EMBL alumna Laura Clarke to pursue a career in AI development
Science is experiencing a paradigm shift as AI continues to transform research, paving the way for breakthroughs. Leveraging the vast amount of life science data openly available to train AI systems is proving to be beneficial for a range of research and biotechnology applications. To find out more, we spoke to EMBL alumna Laura Clarke about how she and her colleagues at BenchSci are using AI to help streamline scientific processes.
Clarke joined EMBL’s European Bioinformatics Institute (EMBL-EBI) as part of the Ensembl project. During her time at EMBL-EBI, she supported the management and delivery of many large-scale sequencing projects. Since leaving EMBL-EBI she has joined BenchSci, where she continues to support scientists using her skills in data management and coordination. Here Clarke discusses her current work at BenchSci and what can be done to enable the future of AI development.
I’m currently working as a Senior Project Manager at BenchSci. BenchSci’s mission is to exponentially increase the speed and quality of life-saving R&D. We do this by providing a software platform, called ASCEND, that is powered by AI that can help scientists identify the best methods and reagents to use for their own research. By helping scientists find this information, BenchSci aims to make experiment design in the lab more efficient.
AI plays a significant role in how ASCEND works. Machine learning algorithms process publications to identify proteins, diseases, antibodies, and other relevant information across all therapeutic areas at different stages of a research project. Scientists can leverage the suite of applications to discover biological connections, surface contextual experimental evidence, define reagents and model systems, and uncover risks early to move the most promising projects forward faster. With ASCEND, scientists can understand what is known, recognise what is yet to be studied, and most importantly, how to execute research plans to make new discoveries.
At EMBL-EBI, I was involved in managing and delivering large-scale sequencing projects, including the 1000 Genomes Project, the Human Cell Atlas (HCA), and the Human Induced Pluripotent Stem Cell Initiative (HipSci).
Initially, I was part of the Vertebrate Genomics Team, but I later moved to the Samples, Phenotypes, and Ontologies Team to help run data coordination services for large-scale projects. Towards the end of my time at EMBL-EBI, my primary focus was on the Human Cell Atlas project, which aims to create comprehensive reference maps of all human cells, to help in understanding human health, and diagnosing, monitoring, and treating disease.
My time at EMBL helped me develop a passion for helping scientists use data. Starting at Ensembl and then expanding into data management, I learned a great deal from the exposure to large-scale projects and the various tools and archives that EMBL supports.
The training I received in management and other areas provided me with a strong skill set that has been useful in defining connections, and guiding the way scientists think about their work.
At EMBL-EBI, I gained valuable experience managing big data and working with scientists, which has allowed me to understand the types of data they need and how they approach their work.
In my current role at BenchSci, I support the bioinformatics and machine learning teams by offering insights from my background. I contribute not only to classic project management tasks such as setting timelines and milestones but also to discussions about problem-solving approaches and the right way to conduct data management. My knowledge of how both academic and industry scientists look at data and plan experiments has been particularly useful.
Open access data and data sharing are essential to AI development because machine learning algorithms require training data. Both for academics and companies, it’s vital to seed innovative ideas with public data. Publicly-labelled data, such as identifying proteins, pathways, or sequences, is valuable as it takes a long time to create and curate. The presence of open access text data and labelled data allows the community to bootstrap ideas and see what works without needing funding or investment before demonstrating an idea’s potential.
At BenchSci, open access data is used extensively. Resources like Ensembl and UniProt are valuable for connecting information and providing ancillary details about proteins and genes. Gene ontology and other resources are also important for building on this knowledge. BenchSci also has access to proprietary databases and contracts with publishers for full-text access that helps us develop our AI platform.
Trustworthiness of AI is a significant challenge. Ensuring the public understands that AI systems, like large language models, do not inherently possess human-like thinking abilities and are primarily designed for pattern recognition and prediction tasks is essential. Trustworthiness becomes even more crucial as AI moves into fields like diagnostics, where bias and accuracy have significant consequences.
It will be exciting to see how we can transition from using AI to uncover existing knowledge to a space where AI can suggest new hypotheses that we haven’t considered before. The speed at which computers can interpret information is much faster than humans, so AI should be able to offer accelerated timeframes for in silico experiments and discoveries. This could help narrow the search space when entering the lab, making experimentation more efficient.
Looking for past print editions of EMBLetc.? Browse our archive, going back 20 years.
EMBLetc. archive