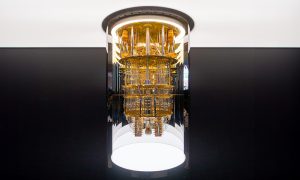
Read the latest Issue
Five takeaways from the recent EMBO | EMBL conference and how AI is making a difference in biology and bioinformatics
By Eva Klimentová, PhD student at Bioinformatics Core Facility, Central European Institute of Technology
Fifteen years ago, machine learning and AI were terms familiar mainly to specialised researchers and industry practitioners. Nowadays, AI is a topic for everyone; it’s in the newspapers, and even our dinner conversations turn to it. We’re living in an age when large language models (LLMs) can chat with us and diffusion models can generate new pictures for us. Biology is evolving to incorporate AI methods as well, employing and adjusting novel techniques for its use in tasks like protein structure prediction or genomic analysis.
This is what brought people from a variety of disciplines to the EMBO | EMBL Symposium ‘AI and biology’ held in a hybrid format from Heidelberg in March 2024. Here are just five takeaways from this dynamic event:
Multimodality in machine learning means integrating diverse input data types (like different imaging techniques, expression profiles, genomic sequences or structures) into one model, and it was one of the most used words at this conference. Multimodality can help us use more diverse samples for machine learning models to learn better and provide a more holistic understanding of mechanisms in biological systems that single-mode data can’t create. One of multimodality modelling’s uses as described during the conference was in cell imaging. However, it might also be particularly useful in medicine– for example, combining genetic information with clinical data that leads to personalised treatments. Using multimodality can also lead to better-designed experiments and show us which modality carries which type of information.
We live in a new world, where LLMs like GPT or Mixtral can change how we think about classical biological or bioinformatics problems. Instead of doing classical gene set analysis by looking at resources like Gene Ontology or the Kyoto Encyclopedia of Genes and Genomes, one can use a dynamic resource. With a bit of prompt engineering, one can directly ask GPT-4 for hypotheses about common gene functions. LLMs can also assist in extracting evidence from the scientific literature to help with tasks such as drug target identification and validation. Another use may be in protein annotation, where LLMs can follow the traditional pipeline by finding the closest homologs and extracting information about them, but in a much shorter time.
When AlphaFold2 came out, it was a real breakthrough in structural biology. It addressed the problem of predicting protein 3D structure from the primary amino acid sequence. However, scientists wanted more than just the tool; they immediately started digging into it to understand its strengths, its limits, and other potential uses.
AlphaFold was originally trained on available protein structures from the Protein Data Bank, which includes around 130,000 experimentally verified 3D structures. Scientists around the OpenFold initiative (open reimplementation of AlphaFold) did some experiments, where they decreased the training dataset all the way down to 1,000 structures. Even this tiny fraction of the original dataset was enough for the model to learn how to predict the 3D structure and it performed better than, for example, the older version of AlphaFold.
Another interesting experiment dealt with fold-switching proteins – proteins with multiple native structures that change their fold based on external factors. When AlphaFold makes a prediction, it first creates a multiple sequence alignment (MSA), where other sequences similar to the input help with modelling the 3D structure. To predict more than one state in the case of fold-switching proteins, we can cluster the input MSA into multiple groups. Each of the groups can be then plugged into AlphaFold separately. This has shown how one can tweak AlphaFold and play with its inputs to predict, for example, multiple states of fold-switching proteins quite accurately.
In cryo-electron microscopy, scientists traditionally aim to reconstruct one static protein structure from a lot of noisy images. However, when focusing on just one structure, we discard approximately 90% of potentially useful data. By using the power of neural networks, it’s now possible to go beyond static snapshots and reconstruct a movie or spectrum of protein structures. This approach captures the molecule’s continuous dynamic behaviour and offers a richer, more detailed understanding of its various states and functions.
A few years ago, AlphaFold basically solved the protein structure prediction challenge. It was an easy-to-understand and well-defined problem, where big companies could enter the biological environment and work on solving it. But as explored during the conference’s panel discussion, big models can start small. It might be enough to define a good biological question that can be answered with data and machine learning. One then has a strong benchmark, which can motivate others to latch onto this scientific question and help the solution progress fast. And that is perhaps what makes AI exciting in biology – the question of how we will harness it next to improve what we can do and what we can learn from it.
AI is a powerful tool that is and will continue to fast-track scientific discovery. By fostering global collaborations, conducting world-class research, and providing pivotal services and tools EMBL is contributing to pushing the boundaries of what’s possible in this rapidly evolving field. It’s essential work that has the potential to revolutionise healthcare, drug discovery, genetics, and many other areas, ultimately leading to significant advancements in improving human and planetary health. Find out more here, and look at other past stories on EMBL’s work in AI and biology.
Looking for past print editions of EMBLetc.? Browse our archive, going back 20 years.
EMBLetc. archive