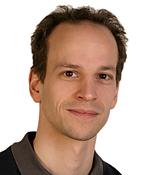
Georg Zeller
Visiting Team Leader (Outgoing)
ORCID: 0000-0003-1429-7485
EditComputational analysis of host-microbiota interactions in disease and drug therapy
Visiting Team Leader (Outgoing)
ORCID: 0000-0003-1429-7485
EditThe human microbiome, the complex ecosystem of microorganisms colonising our body, has increasingly been recognised as an important determinant of human physiology. Modern sequencing technology and computational analysis methodology are now widely used to associate changes in microbiome composition and function with human diseases without any need to culture microbes. However, analysis and interpretation of metagenomic data remains challenging:
To address these challenges, we develop software tools for high-precision profiling of both genomically characterised and unknown microbial species, and the functions encoded in their genomes and transcriptomes. To associate changes in these profiles with various host phenotypes of interest, we have investigated various statistics and machine learning tools and evaluated their applicability to microbiome sequencing data, particularly in the presence of confounding, or in cross-study comparisons. To make these validated statistical and machine-learning tools available to the community, we have published easy-to-use software pipelines. In my group, we focus on the application of this methodology to characterising the gut microbiome in colorectal cancer. For this disease, we have established diagnostic microbiome signatures that are globally validated across seven countries on three continents.