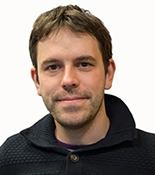
Oliver Stegle
Acting Head of AI centre
ORCID: 0000-0002-8818-7193
EditStatistical genomics and systems genetics
Acting Head of AI centre
ORCID: 0000-0002-8818-7193
EditFind out more about our lab at https://steglelab.org/
How does our genetic background shape phenotypic traits or cause diseases such as cancer? How are genetic and environmental factors integrated at different molecular layers, and how variable are molecular states between individual cells?
We use statistical inference and machine learning as our main tools to address these scientific questions. Growing sample sizes and technological advances demand novel analytical strategies and tools that scale to datasets with millions of observations and account for spatial and temporal dependencies. We develop foundational methods in statistics and machine learning, including efficient parameter inference in models to probe genetic associations and methods for dimensionality reduction.
Computational Genetics
We develop statistical methods to dissect often overlooked dimensions of the genotype-phenotype relationship, including genetic effects of rare variants and indirect genetic effects.
Spatial and Multi-Omics
We develop foundational methods to integrate high-dimensional molecular profiles assayed in bulk or at the single-cell level. This includes innovations to allow for integrating multi-omics readouts across time and space, as well as methods for spatial omics technologies.
Causal Inference Models
We develop methods to model genetic perturbation effects and infer causality. We leverage opportunities provided by engineered perturbations using CRISPR screens combined with single-cell readouts.
We are an interdisciplinary and international team jointly hosted at the European Molecular Biology Laboratory (EMBL) and the German Cancer Research Center (DKFZ) in Heidelberg. In our team we promote openness and collaboration, and value kindness in everything we do. We take pride in every single member and their achievements and are eager to foster their scientific development.
Further Links:
Orcid
Google Scholar
GitHub
ERC INVESTIGATOR