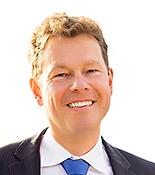
Wolfgang Huber
Group Leader and Senior Scientist
wolfgang.huber [at] embl.org
ORCID: 0000-0002-0474-2218
EditQuantitative Biology and Statistics
Group Leader and Senior Scientist
wolfgang.huber [at] embl.org
ORCID: 0000-0002-0474-2218
EditOur team studies biological systems by developing statistical and computational methods for the analysis of new data types and novel, large systematic datasets. These include single-cell and spatial omics, high-throughput drug- and CRISPR-based perturbation assays, and quantitative imaging. Projects range from applied data analysis for biological discovery to theoretical method development. We study fundamental biological model systems, as well as clinical samples for direct applications in biomedicine and precision oncology. We maintain an extensive network of collaborations. These include the Molecular Medicine Partnership Unit (MMPU) ‘Systems Medicine of Cancer Drugs’, the ERC Synergy project DECODE, the ELLIS unit Heidelberg, and the Bioconductor project.
Our interdisciplinary team comprises researchers from quantitative disciplines – such as physics, mathematics, statistics, computer science – and different fields of biology and medicine. We employ statistics and machine learning to discover patterns in data, understand mechanisms, and to build and investigate models. We pursue three main aims:
Omics and imaging technologies are producing increasingly detailed biology-based understanding of human health and disease. The next challenge is using this knowledge to engineer treatments and cures. To this end, we integrate observational data, such as from large-scale sequencing and molecular profiling, with interventional data, such as from systematic genetic or chemical screens, to reconstruct a fuller picture of the underlying causal relationships and actionable intervention points. A fascinating example is our collaboration on molecular mechanisms of individual sensitivity and resistance of tumors to treatments in our precision oncology project together with Thorsten Zenz at University Hospital Zürich and Sascha Dietrich at University Hospital Düsseldorf.
As we engage with new data types, we aim to develop high-quality computational methods of wide applicability. We consider the release and maintenance of scientific software an integral part of doing science. We contribute to the Bioconductor project, an open source software collaboration to provide tools for the analysis and understanding of genome-scale data. An example is our DESeq2 package for analyzing count data from high-throughput sequencing.
Science is an intellectual adventure and a creative process done by people. Their training and professional development is at the center of what we do. Former group members have moved on to rewarding careers: professors, independent group leaders, senior management or professional scientist roles in industry.
We maintain the textbook Modern Statistics for Modern Biology by Susan Holmes and Wolfgang Huber. The book is available online, for free, as HTML. It was published as a printed book in 2019 by Cambridge University Press.
We run the annual summer school CSAMA—Biological Data Science. It usually takes place in June in Brixen/Bressanone. Here is the webpage of the 2023 edition.
In July 2023, we co-organized the first Biological Data Science Summer School in Ukraine, in Uzhhorod.
We aim to exploit new data types and new types of experiments and studies by developing the computational techniques needed to turn raw data into biology.
ми з україною 🇺🇦 We stand with Ukraine