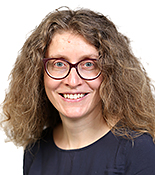
Kreshuk Group
EMBL
Creating synergies between EMBL and Stanford’s research communities
The quest to dissect complex cell states and understand the interplay between genome function and cellular phenotypes underlies many of the ‘big unknowns’ in life sciences today. Rapidly isolating single cells based on a comprehensive range of phenotypes—age, cancerous states, stress levels, specific transcriptional profiles—remains a pivotal challenge. This challenge hinders our capacity to apply functional genomics techniques, like pooled CRISPR screening and single-cell sequencing, to a spectrum of biological questions.
New imaging-enabled cell sorting technologies developed at Stanford and EMBL are poised to revolutionize single cell biology by enabling sorting based on rich morphological phenotypes captured in real-time images. Image-enabled cell sorter (ICS) can acquire high-resolution fluorescence images of millions of single cells per day as they flow through the instrument. However, realizing the full potential of these instruments requires advanced machine learning algorithms to extract biologically-relevant information from the resulting imaging data.
In this project, we will develop cutting-edge deep learning methods to enable sorting of single cells based on any arbitrarily complex morphological phenotype captured by ICS instruments. We will adapt state-of-the-art computer vision models, including self-supervised foundation models trained on the immense scale of imaging data these instruments produce. Our models will expand the insights gleaned from any cell sorting experiment, and through collaboration with industry partners we aim provide real-time morphology-based sorting capabilities by direct integration into instrument software.
To demonstrate the power of this appraoch, we will generate the first image-omics atlas by jointly profiling the transcriptome, epigenome, and morphological phenotypes of thousands of individual cells from multiple cell lines, primary cells, and iPSC-derived cells. We will train deep learning models to predict molecular profiles directly from morphological images, enabling non-destructive isolation of precisely-defined developmental trajectories and cell types.
This work will provide the scientific community unprecedented access to previously intractable cellular phenotypes for diverse applications. Our open-source algorithms and sorted cell populations will drive new discoveries connecting genotypes to complex cellular phenotypes in development, disease, and basic biology.
This project is supported by a Bridging Excellence Fellowship awarded to Jarod Rutledge
Interested in finding out more? Get in touch, we would love to hear from you!